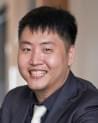
Group Mornin for Healthcare AI
Projects & Demos
Projects with demos
Gout Admission Risk Estimator (demo)
Wang Han, Nur Azizah Allameen, Irwani Ibrahim, Preeti Dhanasekaran, Feng Mengling^, Manjari Lahiri^
To facilitate the development and early institution of targeted interventions to reduce the frequency of gout-related admissions, and potentially allow for the improvement of the care of these complex multimorbid patients, we examined the potential factors that predict the gout-related admissions and come up with a risk prediction model with 5 easy predictors.
Wang Han* and Wesley Yeung*, Angeline Tung, Joey Tay Ai Meng, Feng Mengling#, Shalini Arulanadam#
Our Name Entity Recognition tool for Emergency Medical Service Report (NEREMSR) is an online natural language processing system to identify the potential entities in the paramedics report for auditing purposes.
Mukkesh Kumar, Li Chen, Karen Tan, Li Ting Ang, Cindy Ho, Gerard Wong, Shu E Soh, Kok Hian Tan, Jerry Kok Yen Chan, Keith M Godfrey, Shiao-yng Chan, Mary Foong Fong Chong, John E Connolly, Yap Seng Chong, Johan G Eriksson^, Mengling Feng*^, Neerja Karnani*^
We have developed the first population-centric AI-based GDM risk prediction model for Asian women. The 4 features used in the non-invasive model can be easily measured and assessed during the first trimester (mean arterial blood pressure at booking appointment, maternal age, previous history of GDM and ethnicity). The non-invasive GDM prediction model can be an effective approach to screen and intervene early in women at risk, and also minimize the economic burden of universal testing and GDM associated healthcare in Asian populations.
Machine learning derived prenatal predictive risk model to guide intervention and prevent the progression of Gestational Diabetes Mellitus to Type 2 Diabetes (demo)
Mukkesh Kumar, Li Ting Ang, Cindy Ho, Shu E Soh, Kok Hian Tan, Jerry Kok Yen Chan, Keith M Godfrey, Shiao-yng Chan, Yap Seng Chong, Johan G Eriksson^, Mengling Feng*^, Neerja Karnani*^
We developed a machine learning model for early prediction of postpartum Type 2 Diabetes (T2D) following routine antenatal Gestational Diabetes Mellitus (GDM) screening. The prediction model for postpartum T2D, comprises of 2 mid-gestation features (mid-pregnancy BMI after gestational weight gain; diagnosis of GDM). Mid-gestation weight gain effects combined with the metabolic derangements underlying GDM during pregnancy signal future T2D risk in Singaporean women. The state-of-the art machine learning model can be leveraged as a rapid risk stratification tool during prenatal care.
Automated Machine Learning (AutoML) derived preconception predictive risk model to guide early intervention for Gestational Diabetes Mellitus (demo)
Mukkesh Kumar, Li Ting Ang, Hang Png, Maisie Ng, Karen Tan, See Ling Loy, Kok Hian Tan, Jerry Kok Yen Chan, Keith M Godfrey, Shiao-yng Chan, Yap Seng Chong, Johan G Eriksson^, Mengling Feng*^, Neerja Karnani*^
We developed a machine learning model for early prediction of Gestational Diabetes Mellitus (GDM) during preconception among women in Singapore. A stacked ensemble model with gradient boosting classifier and linear support vector machine classifier (stochastic gradient descent training) was derived using genetic programming, achieving an excellent AUC of 0.93 based on four features [glycated hemoglobin A1c (HbA1c), mean arterial blood pressure, fasting insulin, triglycerides/HDL ratio]. Our trained predictor is deployed as a web application that can be easily employed in GDM intervention programs, prior to conception.
Address
12 Science Drive 2, Singapore 117549
ephfm{at}nus{dot}edu{dot}sg or mornin{at}gmail{dot}com
Mengling Feng @ MIT, US © 2017